Enhancing Business Efficiency through Machine Learning for Systems
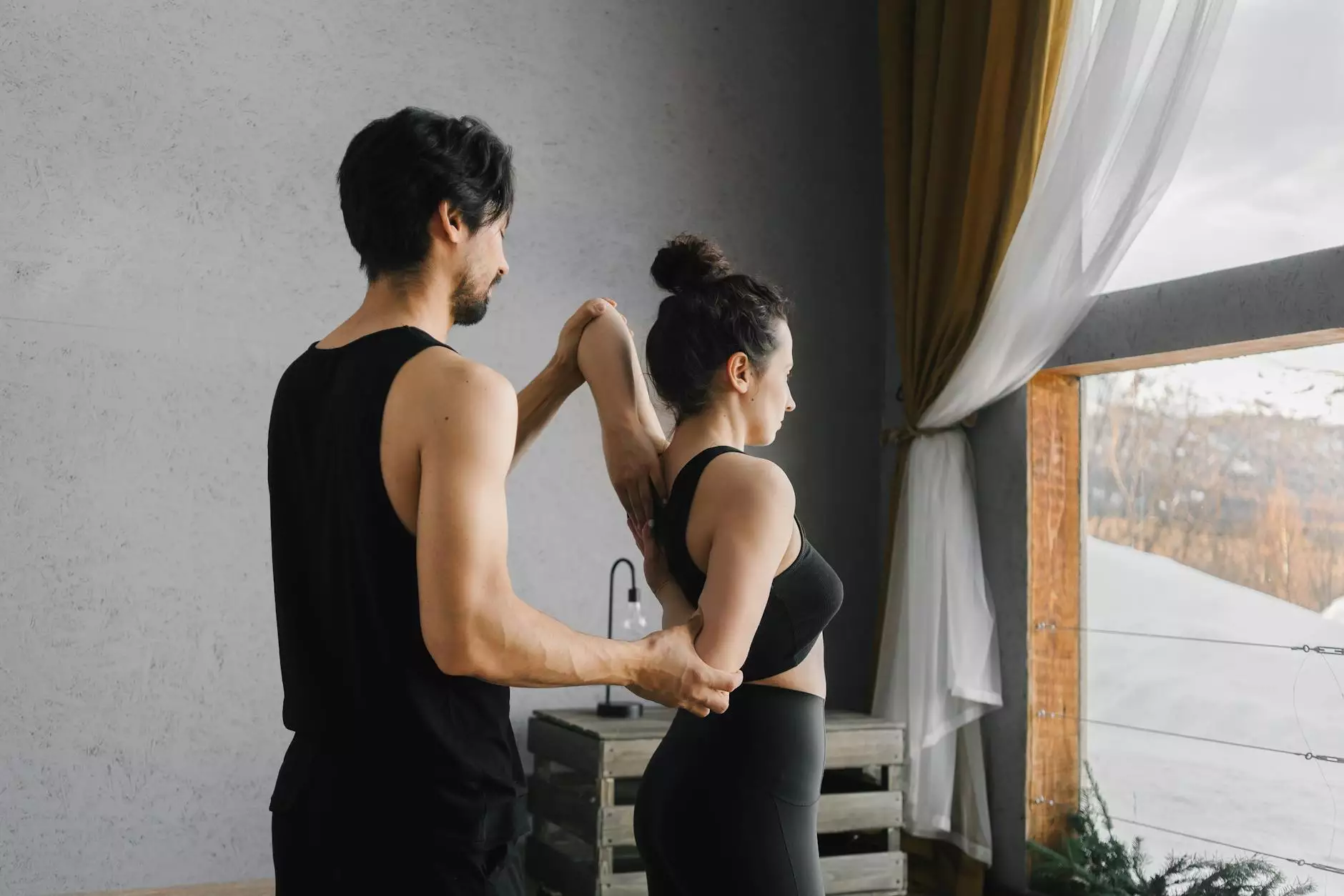
In the rapidly evolving business landscape, the integration of technology is not merely an advantage; it has become a necessity. Among the plethora of technological advancements, machine learning stands out as a transformative force. By leveraging machine learning for systems, businesses can optimize operations, enhance decision-making processes, and achieve unprecedented levels of efficiency. In this comprehensive overview, we’ll delve into the multifaceted benefits of machine learning, particularly within the realms of content management services, business process automation services, and data governance systems.
Understanding Machine Learning for Systems
Machine learning is a subset of artificial intelligence that enables systems to learn from data, identify patterns, and make decisions with minimal human intervention. This capability is particularly beneficial for businesses aiming to streamline operations and improve their offerings. By integrating machine learning into their systems, organizations can harness the power of data in new and innovative ways.
Key Concepts in Machine Learning
- Algorithms: Techniques that process data to learn patterns and make predictions.
- Data Input: The information fed into the algorithms to facilitate learning.
- Training and Testing: The process of using a subset of data to train the machine learning model and another subset to test its accuracy.
Benefits of Machine Learning in Business
As businesses adapt to more complex environments, the need for intelligent systems grows. Here’s how adopting machine learning for systems can benefit your organization:
1. Enhanced Decision-Making
Machine learning models analyze vast amounts of data to generate insights that inform strategic decisions. Businesses can predict trends, assess risks, and identify opportunities with greater accuracy than conventional methods. For instance, utilizing predictive analytics, companies can forecast sales trends, enabling proactive decision-making regarding inventory and staffing.
2. Improved Operational Efficiency
Automating routine tasks with machine learning greatly enhances operational efficiency. By identifying and streamlining repetitive processes, organizations can reduce costs and direct their human resources towards innovation and customer service. For example, a machine learning-driven business process automation service can handle invoicing, freeing up employees for more strategic work.
3. Elevated Customer Experience
Personalization is key in today’s competitive market. Machine learning enables businesses to analyze customer data and tailor offerings to individual preferences, thus enhancing the overall customer experience. This is particularly evident in e-commerce, where algorithms can suggest products based on browsing history and preferences.
4. Data Governance and Compliance
With increasing regulatory scrutiny, effective data governance systems are essential. Machine learning helps organizations manage data integrity and compliance risks by monitoring and analyzing data usage patterns, subsequently enforcing policies to safeguard sensitive information.
Implementing Machine Learning for Systems
Integrating machine learning into your business processes requires careful planning and execution. Here are the essential steps to successfully implement machine learning:
Step 1: Define Objectives
Before embarking on a machine learning project, clarify what you aim to achieve. Set measurable goals, whether it’s reducing operational costs, improving customer satisfaction, or enhancing data management.
Step 2: Data Collection and Preparation
Quality data is the cornerstone of any machine learning project. Collect relevant data from various sources, ensuring it is clean, organized, and representative of what you wish to analyze.
Step 3: Choose the Right Algorithms
Depending on your objectives, select the most suitable machine learning algorithms. This could range from supervised learning for predictive tasks to unsupervised learning for clustering data without prior labeling.
Step 4: Train and Validate the Model
Divide your data into training and testing sets. Train your model using the training set, then validate its performance using the testing set. Adjust parameters as necessary to optimize outcomes.
Step 5: Implement and Monitor
Deploy the machine learning model within your systems. Continuous monitoring is essential to ensure the model adapts over time and remains relevant as new data and conditions arise.
Case Studies: Successful Machine Learning Implementations
Numerous businesses have successfully harnessed machine learning for systems. Here are a few notable case studies:
Case Study 1: Retail Industry
A leading retailer implemented a machine learning algorithm to analyze customer buying patterns. This initiative resulted in a 25% increase in sales due to improved product recommendations and targeted marketing campaigns.
Case Study 2: Financial Services
A major bank utilized machine learning to enhance its fraud detection system. By processing transaction patterns, the bank was able to reduce fraudulent activity by 40%, safeguarding customer assets and enhancing trust.
Case Study 3: Healthcare
A healthcare provider used machine learning to predict patient admissions and optimize staffing. This predictive model reduced wait times and improved patient care, leading to a 30% increase in patient satisfaction scores.
The Future of Machine Learning in Business
The potential of machine learning for systems is vast. As technology advances and data becomes more accessible, businesses will increasingly rely on machine learning to drive innovation and enhance operational effectiveness. Emerging trends include:
1. Increased Automation
More organizations will adopt machine learning-driven automation tools, allowing for quicker responses to market changes and customer demands.
2. Enhanced Predictive Analytics
As algorithms improve, predictive analytics will become more accurate, enabling businesses to anticipate market trends and consumer behavior with greater precision.
3. Ethical AI
With the proliferation of AI technologies, there will be a heightened emphasis on ethical considerations, ensuring that machine learning applications are transparent and equitable.
Conclusion
In conclusion, embracing machine learning for systems is a profound step towards enhancing business operations and staying competitive in an ever-changing environment. By leveraging this transformative technology across content management services, business process automation services, and data governance systems, organizations can unlock new efficiencies, improve decision-making, and foster greater innovation.
For businesses looking to lead in this digital era, integrating machine learning is not just an option; it is a pathway to success. By investing in these advanced technologies, companies can position themselves for enduring growth and resiliency in the future.